Introduction
Ann Kimball and John W. Johnson Center for Cellular Therapeutics at Houston Methodist
Houston Methodist Dr. Mary and Ron Neal Cancer Center
The Food & Health Alliance within the Houston Methodist Lynda K. and David M. Underwood Center for Digestive Disorders, Immunology Center and the Fondren Inflammation Collaborative
Houston Methodist Cockrell Center for Advanced Therapeutics
Paula and Joseph C. “Rusty” Walter III
Translational Research Initiative
Jerold B. Katz Academy of Translational Research
Infectious Diseases Research Fund
George and Angelina Kostas Research Center for Cardiovascular Medicine
New Endowed Chairs Positions
EnMed
Center for Bioenergetics
result
Clinical Research
Outcomes, Quality and Healthcare Performances
Restorative Medicine
Precision Medicine
Science in Service
of
Medicineresult
President's letter
2022 Metrics
Cycle of Translation
Visionary Gifts of Hope
Introduction
Ann Kimball and John W. Johnson Center for Cellular Therapeutics at Houston Methodist
Houston Methodist Dr. Mary and Ron Neal Cancer Center
The Food & Health Alliance within the Houston Methodist Lynda K. and David M. Underwood Center for Digestive Disorders, Immunology Center and the Fondren Inflammation Collaborative
Houston Methodist Cockrell Center for Advanced Therapeutics
Paula and Joseph C. “Rusty” Walter III Translational Research Initiative
Jerold B. Katz Academy of Translational Research
Infectious Diseases Research Fund
George and Angelina Kostas Research Center for Cardiovascular Medicine
New Endowed Chairs Positions
EnMed
Center for Bioenergetics
From Discovery to Clinic
What is "Discovery to Clinic"?
Clinical Research
Houston Methodist Conducts First-Ever Study into a Challenging Situation
Can Regulating Cellular Aging Mitigate Both Cancer and Heart Disease?
Innovative Treatment for Chronic Rhinitis is Safe and Effective
Masters of Disguise: Glioblastomas Trick the Immune System by Masquerading as Reproductive Tissue
Improved Options for Patients with Severe Retinal Vascular Disease
A New FDA-Approved Treatment for Sufferers of Chronic Constipation
Houston Methodist joins the Gulf Coast Consortia
Outcomes, Quality and Healthcare Performance
New Findings on RNA Helicases May Yield New Intestinal Disease Therapy
Houston Methodist and Pennsylvania State University Collaborate on a Smartphone App That Could Revolutionize Stroke Diagnosis
New Frontiers to Improve Cardiovascular Medicine and Disease Management
Ongoing Lessons in a Pandemic
Transplants can Boost Survival Rate of Patients with Unresectable Liver Cancers
Telehealth Video Visits During the COVID-19 Pandemic – a Glimpse into the Future?
SARS-CoV-2 Induced Chronic Oxidative Stress and Endothelial Cell Inflammation May Increase Likelihood of Cardiovascular Diseases and Respiratory Failure
Restorative Medicine
Lessening Pain After Knee Replacement Surgery
Do Motor Neurons First Die in the Brain? Study Provides Clues about ALS Origins
Bringing Back Hand Function in People with Complete Spinal Cord Injury
Novel Vascular Engineering Platforms Are a Boon for Bioengineering
Ultra-high-Resolution Scanner Reveals if Knee Injury Advances to Osteoarthritis
Houston Methodist Model Demonstrates Reversal from Heart Failure State, Creating the Potential for Innovative Treatment Avenues
Precision Medicine
Rapidly Scalable, All-Inducible Neural Organoids Could Facilitate Drug Screening for Neurological Diseases
Importance of the Coronary Artery Calcium Score in Risk Assessment and Prevention of Atherosclerotic Cardiovascular Disease
COVID-19 Infection in Crucial Brain Regions May Lead To Accelerated Brain Aging
Interleukin 9 Secreting Polarized T Cells Show Potential in Solid and Liquid Tumor Treatment
The NanoLymph: Implantable. Adaptable. Anti-cancer
Discovery to Clinic
Outcomes, Quality and Healthcare Performance
Houston Methodist and Pennsylvania State University Collaborate on a Smartphone App That Could Revolutionize Stroke Diagnosis
Houston Methodist and Pennsylvania State University Collaborate on a Smartphone App That Could Revolutionize Stroke Diagnosis
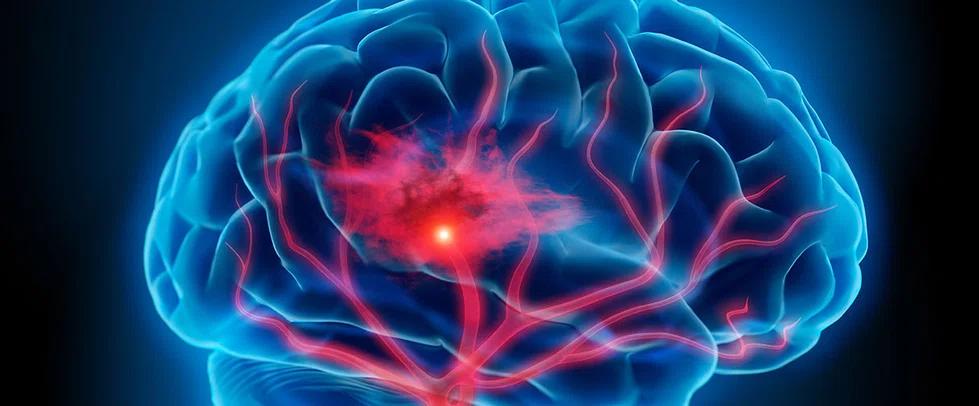
Diagnosing a stroke quickly in the Emergency Department (ED) is crucial. With each passing minute that a stroke goes undiagnosed and treated, millions of neurons are lost, and the time for providing the best treatment options available passes. Severe strokes are obvious for physicians to diagnose, but often, patients exhibit minor to moderate stroke symptoms that can stem from stroke or other causes. If missed, these patients can end up waiting longer for an accurate diagnosis, thus delaying treatment and possibly missing an opportunity to receive optimal treatment.
The most effective diagnostic tool for ischemic stroke is the MRI, but it is limited by patient cooperation, prolonged turn-around time and delaying treatment. So, it is not often used as a first line test in the ED. CT scans are simple to perform but only show definite strokes, and they will miss early changes and minor strokes. Also, for patients in whom stroke is not suspected, no stroke investigations will be performed. Neurologists can effectively use physical exam and history-based scores for triage in stroke diagnosis, but these scores lack accuracy and require widespread training. And while it would be ideal, it is not always possible to have a neurologist evaluate a patient in the ED.
An Intelligent Augmented Lifelike Avatar App
for Virtual Physical Examination of Suspected Strokes
To meet this diagnostic challenge, John J. Volpi, MD, Director, Eddy Scurlock Stroke Center and Associate Professor of Clinical Neurology, and his team developed an effective and rapid stroke diagnostic tool.
At the 2021 Annual International Conference of the IEEE Engineering in Medicine and Biology Society, the Houston Methodist collaborative group of Volpi and Stephen T.C. Wong, John S. Dunn Presidential Distinguished Chair in Biomedical Engineering and Professor of Computer Science & Bioengineering in Oncology, presented their work on a novel smartphone app developed in collaboration with researchers at Pennsylvania State University. The app uses a machine learning algorithm for computer-aided evaluation of facial movement weaknesses and speech in patients to determine the presence of stroke; it emulates the ED triage process using a smartphone.
The app is an intelligent-augmented lifelike avatar mobile app (iLAMA) designed to automate and streamline the NIH Stroke Scale physical examination by integrating computer vision and sensor readings. Designed for elderly patients, the app features an animated 3D model of a physician who guides the user through the exam consisting of five core tasks. The first two involve rolling the eyes to the left and then right, and then smiling as wide as possible. The app identifies facial landmarks and analyzes facial palsy. In the next task, the user extends the arm, holding the phone at shoulder level so the phone’s gyroscope can detect acceleration to determine possible arm weakness. Then the app tracks location of the hand key points and looks for ataxia-based accuracy of the touch locations. Finally, using an accelerometer, the app calculates the user's forward acceleration while walking and detects possible imbalances. The analyzed results of these tasks are then sent to a specified neurologist or stroke specialist for review.
To train the AI model, the researchers created a dataset from more than 250 Houston Methodist patients experiencing stroke symptoms. Using an iPhone to record the encounter, each patient performed a test to demonstrate their speech and communication. The results of the study were highly significant. Their app was 93% correct in detecting a possible stroke compared to the ED physician rate of 71%, and the app was also better at avoiding overdiagnosis with an accuracy of 79% compared with the physician’s rate of 73%. Of note, the app encounter took less than five minutes and did not include any additional data, such as scan results or the physician’s prior history.
This rapid stroke assessment app will allow expedited diagnosis of stroke by physicians, and faster delivery of the most effective treatment options. Future versions of the app are already under development to give those at higher risk for stroke, and the ability to perform a self-assessment immediately upon symptom presentation by their caregivers. Ultimately, this would further reduce the time from stroke onset to effective treatment and significantly improve patient outcomes, increasing the chances of survival and recovery.
By combining an AI tool with the emergency room physician’s clinical knowledge, this “augmented intelligence” innovation will be a key advantage to preventing and/or surviving a stroke.