clinical research
Predicting the Future of Cancer Treatment
Non-small cell lung cancer (NSCLC), named for the appearance of its cancer cells under a microscope, is the most common (85%) of all lung cancers.
The primary risk factor for NSCLC is smoking. The longer the exposure to smoking, the higher the risk. Available treatments include most standard options though development of resistance to platinum-based chemotherapies reduces efficacy resulting in relapses and patient mortality.
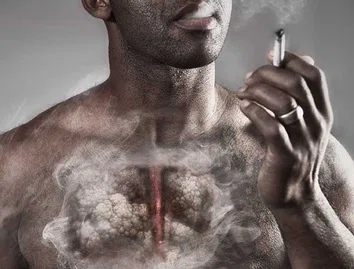
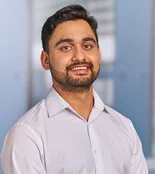
Prashant Dogra, PhD
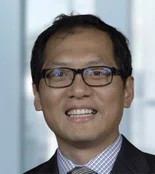
Zhihui Wang, PhD
Recently, the overexpression of microRNA-155 (miR-155) in tumor cells has emerged as a key contributor to chemoresistance and is associated with increased NSCLC aggressiveness and unfavorable prognoses. Houston Methodist’s Mathematics in Medicine faculty Prashant Dogra, PhD and Zhihui Wang, PhD, have been using mathematical modeling to further investigate the role of miR-155 in NSCLC.
Dogra’s expertise spans multiscale mechanistic modeling, quantitative systems pharmacology, pharmacometrics, and their integration in interdisciplinary basic and translational research for drug development and treatment optimization. He utilizes multimodal experimental and clinical data to leverage machine learning and develop predictive models to study disease dynamics, support drug development, address drug delivery challenges, and identify optimal treatment strategies for enhanced therapeutic efficacy and safety.
Wang is an expert at the forefront of computational biology, modeling-aided drug target discovery, biophysical modeling of drug transport and multiscale cancer modeling.
The team’s recent work on miR-155 not only reveals new details about miR-155-induced therapeutic resistance, but also demonstrates the potential of pre-clinical predictive models to predict clinical efficacy.
The multiscale mechanistic model, calibrated with in vivo data, can serve as a valuable tool for predicting clinical treatment endpoints and guiding personalized treatment strategies for systemic therapies. These results offer a foundation for future clinical trials of anti-miR-155— bringing us closer to more effective and safer therapeutic approaches for NSCLC patients.
Prashant Dogra, PhD
Assistant Research Professor of Mathematics in Medicine
Elevated miR-155 expression in NSCLC promotes cisplatin resistance and negatively impacts treatment outcomes. However, miR-155 can also boost anti-tumor immunity by suppressing PD-L1 expression. Dogra’s group previously identified a novel mechanism by which miR-155 induces resistance to cisplatin through a TP53-mediated feedback loop. More recently, they developed a multiscale mechanistic model, calibrated with in vivo data and then extrapolated to humans, to investigate the therapeutic effects of nanoparticle-delivered anti-miR-155 in NSCLC, alone or in combination, with standard-of-care drugs.
Previous modeling efforts focused on the molecular effects of miRNAs on tumor growth dynamics, but lacked the inclusion of a drug delivery system, its associated pharmacokinetics, and the transport phenomena necessary to assess the anti-cancer therapeutic efficacy of miRNAs or their antagonists. To address this, the team developed a multiscale model of tumor growth dynamics integrated into a pharmacokinetic model to assess the translational potential of miR-22 in triple-negative breast cancer. In their most recent study, they extended this modeling work to further incorporate tumor-immune interactions and related molecular effects of miR-155 to evaluate the translational potential of anti-miR-155 in early-stage NSCLC—both alone and in combination with standard-of-care drugs. The model was rigorously calibrated with in vivo datasets from NSCLC-bearing mice, followed by interspecies scaling to humans through allometric scaling techniques, and extrapolated to humans for translationally relevant simulations and analyses.
The study provides strong quantitative rationale for using anti-miR-155 therapy to treat NSCLC. Results of the drug combination studies underscore the potential for synergy, but also highlight the potential for antagonism. The modeling efficacy demonstrates the utility of using preclinical data to predict clinical trial success, a tool that could help refine which potential therapeutics make it that far.
“The multiscale mechanistic model, calibrated with in vivo data, can serve as a valuable tool for predicting clinical treatment endpoints and guiding personalized treatment strategies for systemic therapies,” said Dogra. “These results offer a foundation for future clinical trials of anti-miR-155, bringing us closer to more effective and safer therapeutic approaches for NSCLC patients.”
November 2024
Related Articles
Share this story