outcomes research
Future of Personalized
Cancer Medicine
Future of Personalized
Cancer Medicine
Mathematical modeling of cancer immunotherapy
can help optimize personalized medicine.
Mathematical modeling of cancer immunotherapy
can help optimize personalized medicine.
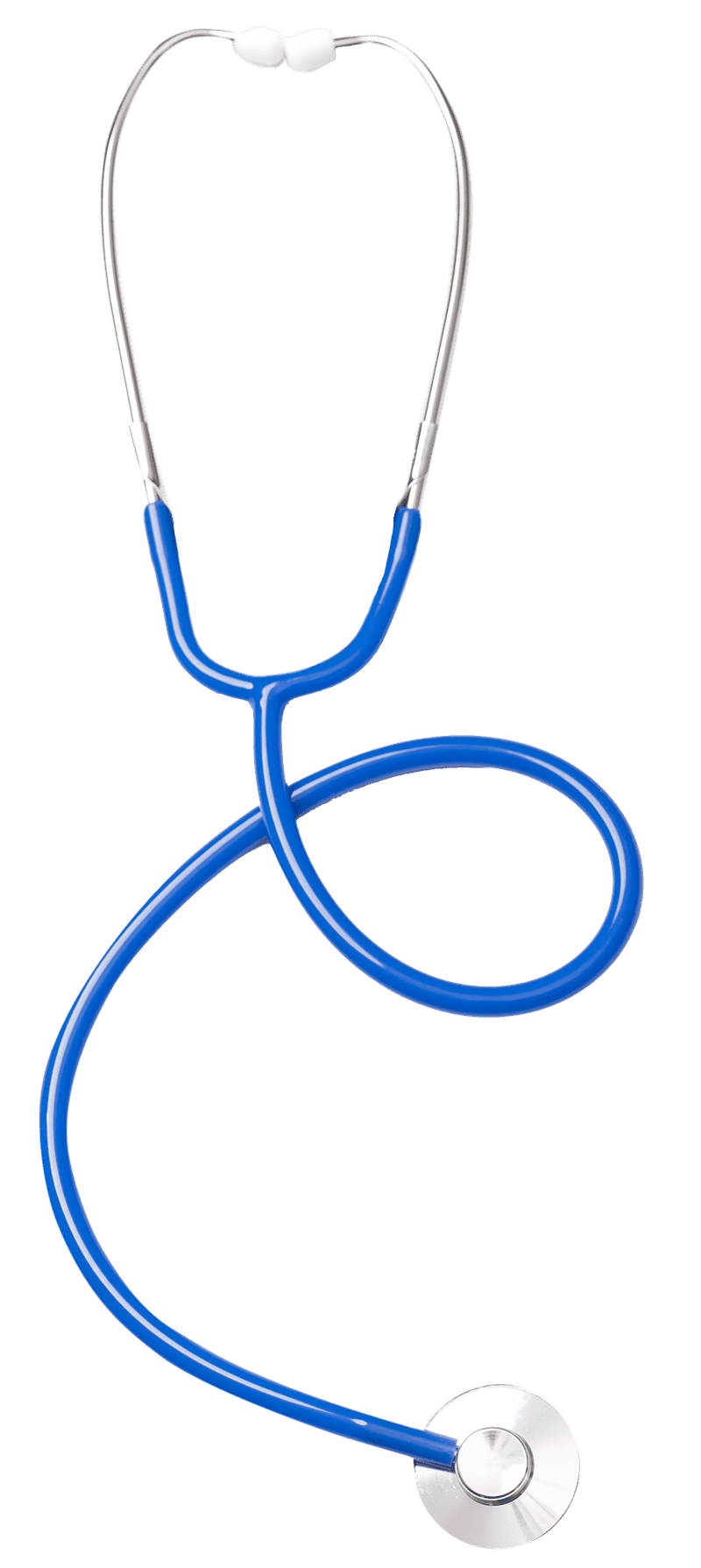
Mathematical modeling has many applications in the field of biomedical research, including a better understanding of disease pathophysiology at the molecular level and predicting treatment outcomes. Zhihui Wang, PhD, Professor of Computational Biology in Medicine, along with Joseph Butner, PhD, previously Instructor in Computational Biology published a review study in 2022 Nature Computational Science that details how mathematical modeling of cancer immunotherapy can have a significant positive impact on personalized medicine. This review study specifically discusses cancer immunotherapy modeling — its applications and challenges.
Oncology is a rapidly evolving field of modern medicine owing to technological advances in human physiology, anatomy, epidemiology and other related areas. However, despite the technological advances and the state-of-the-art treatments available, cancer continues to be a leading cause of death globally and a source of enormous economic burden. According to the World Health Organization—one in six deaths is due to cancer and about 10 million cancer-related deaths occurred in 2020. Efficient collaborations between clinicians and computational biologists can enable advances in mathematical modeling to be correctly applied to reduce this global disease burden.
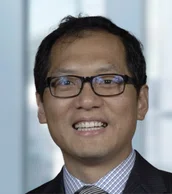
Zhihui Wang, PhD
Joseph Butner, PhD
Typical cancer immunotherapy methodologies include immune checkpoint inhibitor therapy, adoptive cell transfer therapy, vaccination, and exogenous cytokine therapy. However, cancer immunotherapy can also be used in combination with traditional cancer therapeutics such as chemotherapy and radiation therapy. Combination therapies typically lead to better treatment outcomes as compared to monotherapies since multiple key pathways are targeted synergistically.
To date, there are more than 600 cancer drugs including at least 30 immunotherapy agents that are approved by the Food and Drug Administration (FDA). This indicates a high number of possible drug combinations with unknown treatment outcomes. Notably, cancer drug development is a highly costly and time-consuming process. Combination therapies can be cost-effective since many of the drugs are already FDA-approved. Also, it is not possible to systematically assess each combination via clinical trials alone. Hence, mathematical modeling combined with artificial intelligence may prove to be indispensable to efficiently and effectively identify optimal drug combinations as well as predicting treatment outcomes.
Typical cancer immunotherapy methodologies include immune checkpoint inhibitor therapy, adoptive cell transfer therapy, vaccination, and exogenous cytokine therapy. However, cancer immunotherapy can also be used in combination with traditional cancer therapeutics such as chemotherapy and radiation therapy. Combination therapies typically lead to better treatment outcomes as compared to monotherapies since multiple key pathways are targeted synergistically.
To date, there are more than 600 cancer drugs including at least 30 immunotherapy agents that are approved by the Food and Drug Administration (FDA). This indicates a high number of possible drug combinations with unknown treatment outcomes. Notably, cancer drug development is a highly costly and time-consuming process. Combination therapies can be cost-effective since many of the drugs are already FDA-approved. Also, it is not possible to systematically assess each combination via clinical trials alone. Hence, mathematical modeling combined with artificial intelligence may prove to be indispensable to efficiently and effectively identify optimal drug combinations as well as predicting treatment outcomes.
We expect that engineered, computation-based immunotherapy treatment strategies will become a critical part of the next-generation therapies by facilitating clinical translation of new drugs and optimizing personalized treatment strategies for maximized therapeutic success. We believe that such applications will include identifying new biomarkers, utilizing systems approaches to expedite drug development and deployment pipelines, and enabling clinical design of personalized treatment strategies. If the modeling field is to achieve its stated goal of clinical adoption and improvement of personalized treatment strategies, the modelers must recognize that they are designing for a customer: the physician, patient or wet-lab drug developer. We hope that computational scientists will continue to strive for more collaborative efforts with physicians and oncologists to bridge this gap and increase the likelihood of translating their modeling work to the clinic.
Zhihui Wang, PhD
Professor of Computational Biology in Medicine
Joseph Butner, PhD
Previously Instructor in Computational Biology
Butner noted,” If the modeling field is to achieve its stated goal of clinical adoption and improvement of personalized treatment strategies, the modelers must recognize that they are designing for a customer: the physician, patient or wet-lab drug developer.” He continued, "We hope that computational scientists will continue to strive for more collaborative efforts with physicians and oncologists to bridge this gap and increase the likelihood of translating their modeling work to the clinic.”
Personalized cancer medicine can reduce cancer mortality when tailored strategies make cancer treatments more effective and have fewer side effects. In this regard, mathematical modeling can lead to a better understanding of cancer immunology and immunotherapy which is crucial for accurate personalized modeling predictions. It can also help in unraveling the molecular basis of personalized cancer treatments, genetic changes occurring inside tumors, nuances of targeted therapies, and personalized pharmacogenomics when combined can significantly improve the future of personalized cancer medicine.
Joseph Butner, Prashant Dogra, Caroline Chung, Renata Pasqualini, Wadih Arap, John Lowengrub, Vittorio Cristini, Zhihui Wang. Mathematical modeling of cancer immunotherapy for personalized clinical translation. Nat Comput Sci 2, 785–796 (2022).
https://doi.org/10.1038/s43588-022-00377-z
Abanti Chattopadhyay, PhD
December 2023
Related Articles