Follow us
Copyright 2024. Houston Methodist, Houston, TX. All rights reserved.
Outcomes Research
App Reduces Risk of Falls
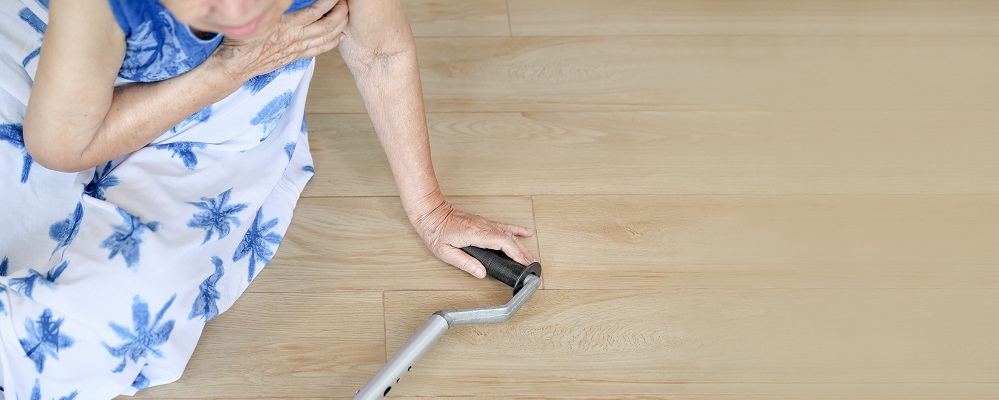
App uses AI to identify patients at highest risk for severe falls
Research faculty and hospital systems teams worked together to develop an app to prevent falls by identifying patients at high risk for falling.
In 2015, the estimated medical costs attributable to fatal and nonfatal falls came to approximately $50 billion nationwide. For nonfatal falls, Medicare paid approximately $28.9 billion, Medicaid $8.7 billion, and private and other payers $12 billion. Overall medical spending for fatal falls was estimated to be $754 million.
In response, Houston Methodist took on the challenge of reducing falls for patients at highest risk. Stephen Wong, PhD, John S. Dunn, Sr. Presidential Distinguished Chair in Biomedical Engineering, worked with Mark Vassallo, the hospital system's vice president of quality, to develop an app using artificial intelligence to predict risk and prevent severe patient falls. The results were published in a study entitled, “Preventing Inpatient Falls with Injuries using Integrative Machine Learning Prediction: A Cohort Study,” in npj Digital Medicine in December 2019.
The research used a machine learning app aimed at preventing patients from severe fall-related injuries and deaths. This AI technology was developed and tested over an eight-month period to help address the growing concern of severe patient falls with seniors and the worry it causes their care-providers and caregivers.
The AI app predicts the risk of injury when a patient falls. Clinical parameters and patient demographics, such as bone density, diagnosis and past procedures, are used to populate the app, which then triggers tailored interventions to prevent these high-risk severe injury patients from falling – whether they are in the hospital setting or with home caregivers.
This AI technology can be integrated into the patient’s electronic medical record (EMR), automatically flagging and alerting the care-providers to patients for high-risk fall with harm when they enroll in the hospital. This will then trigger a prevention-focused intervention plan or clinical care path, making processes and safety measures easier for clinicians to implement. The immediate benefit to the patient is to avoid falling and injuries, or even death. The benefit for the hospital is the ability to avoid additional costs and/or lawsuits for these types of patients.
Wang L, Xue Z, Ezeana CF, Puppala M, Chen S, Danforth RL, Yu X, He T, Vassallo ML & Wong STC. Preventing inpatient falls with injuries using integrative machine learning prediction: a cohort study. 2019 Dec 12, npj Digit. Med. 2, 127, DOI:10.1038/s41746-019-0200-3.
Gale Smith, December 2019